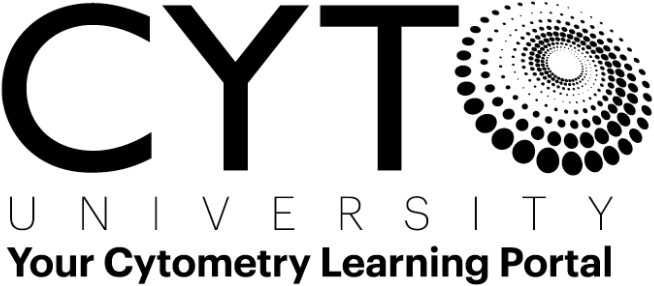
Collaborative Image Analysis in the Cloud for Improved Reproducibility and High Scalability
Recorded On: 12/10/2020
-
Register
- Visitor - $50
- Bronze - Free!
- Silver - Free!
- Gold - Free!
- Platinum - Free!
- Community Administrator - Free!
- ISAC Staff - Free!
- Bronze Lab Membership - Free!
- Silver Lab Membership - Free!
- Platinum Lab Membership - Free!
About the Presenters
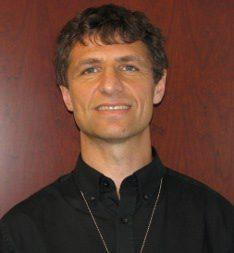
Dr. Peter Bajcsy
Project Lead
National Institute of Standards and Technology (NIST)
Information Technology Laboratory (ITL)
Peter Bajcsy received his PhD in electrical and computer engineering in 1997 from the University of Illinois at Urbana-Champaign and an MS in electrical and computer engineering in 1994 from the University of Pennsylvania. He worked for machine vision, government contracting, and research and educational institutions before joining National Institute of Standards and Technology (NIST) in June 2011. At NIST, he is leading a project focusing on the application of computational science in metrology, specifically live cell and material characterization at very large scales.
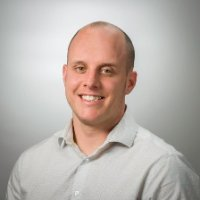
Dr. Nathan Hotaling
Vice President
Data Science Solutions
Information Technology Research Branch
National Center for Advancing Translational Science-NIH
Dr. Nathan Hotaling is a senior data scientist within the Information Resources Technology Branch at National Center for Advancing Translational Science-NIH (NCATS). He received his PhD in biomedical engineering from the Georgia Institute of Technology and an MS in clinical research from Emory University. After his PhD, Nathan conducted postdoctoral research in a joint project between the National Institute of Standards and Technology (NIST) and the National Eye Institute (NEI), where he began to develop a platform to analyze high-content image datasets collected for cell bio-manufacturing. This work led to his transition to his current position where he oversees the development of a scalable image analysis platform to non-invasively assess cell and tissue architecture, functionality, phenotype, consistency, and viability. Using this platform with novel machine learning and deep learning techniques, he intends to unlock the next “-omics” of cell analysis, "Vis-omics," for both research and clinical projects. He has co-authored 22 journal papers, two book chapters, and three patents.
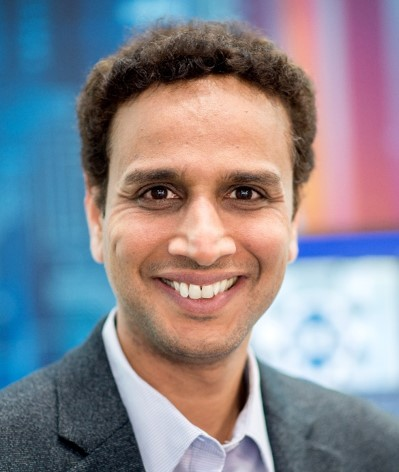
Dr. Sreenivas Bhattiprolu
Head of Digital Solutions
Research Microscopy Solutions
ZEISS
Dr. Sreenivas Bhattiprolu (Sreeni) is the head of digital solutions at Carl Zeiss Microscopy. His team focuses on solving tough microscopy challenges by leveraging the latest advancements in digital technology and artificial intelligence. Sreeni has over 25 years of experience in microscopy in a variety of fields including life sciences, materials sciences, geosciences, electronics, and semiconductor technologies. Sreeni received his PhD in materials sciences and engineering from Michigan Technological University and earned his master’s degree in physics from the University of Hyderabad.
Webinar Summary
According to a recent survey by Nature, more than 70 percent of researchers have tried and failed to reproduce another scientist's experiments. Many factors contributing toward irreproducibility can be addressed via automation and collaborative work. Computer cloud provides the right infrastructure to automate image analysis tasks, especially for resource-intensive applications. Cloud's importance in regards to collaborative work has also been heightened in light of the COVID-19 pandemic due to its accessibility from any location and any networked device. This webinar further explains the benefits of cloud-based image analysis and introduces the audience to two platforms that facilitate automation and collaborative work with microscopy images. These two platforms have been independently developed by ZEISS and NIST/NIH, respectively. The presentation will go over the main features of the two platforms that run computational workflows formed by software containers that are interoperable. The discussion will include a variety of commercial and open source aspects in developing and using such platforms by the ISAC community.
Learning Objectives
- Why web/cloud-based image software solutions? How does your software work?
- What are the advantages/disadvantages of your platform compared to traditional/existing approaches? When is best to think about using WIPP or APEER to solve quantitative imaging problems?
- What does your software do? What functionality does it have? What is the best way for a potential user or group to get started using your platform (i.e., downloading, required hardware, necessary expertise, etc.)?
- Considering that web/cloud-based platforms are a newer tool with a much smaller user population compared to packages such as ImageJ, Matlab, and Cell Profiler, what do you see as the future for these platforms? How will they grow? What are your plans for rolling out your platform and increasing adoption rates?
CMLE Credit: 1.0