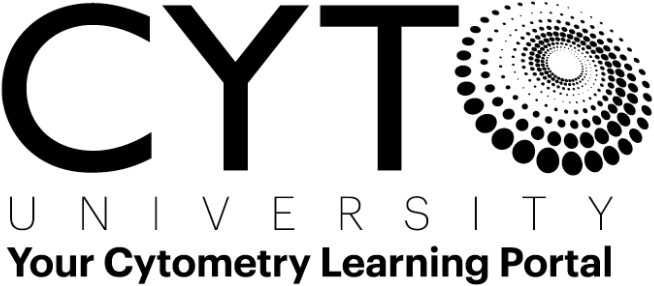
CYTO 2019
-
Register
- Visitor - $50
- Bronze - Free!
- Silver - Free!
- Gold - Free!
- Platinum - Free!
- Community Administrator - Free!
- ISAC Staff - Free!
- Bronze Lab Membership - Free!
- Silver Lab Membership - Free!
- Platinum Lab Membership - Free!
Spectral Unmixing and Compensation in Flow and Image Cytometry
Genomic Cytometry: Using Mulitomic Approaches to Increase Dimensionality in Cytometry
Data-Mining Techniques for Single Cell Data
Current Standards in Flow Cytometry Cell Sorter Biosafety
Photodetection in Flow Cytometry: Key Considerations for the Latest Detectors Including High QE PMTs, APDs and 3rd Generation SiPMs
Cell Image Classification: An Overview of Methods with Software Examples
Flow Cytometry of Extracellular Vesicles: Guidelines for Reporting Methods and Results
State-of-the-Art Lectures - Translating a Trillion Points of Data into Therapies, Diagnostics and New Insights into Immunology
State-of-the-Art Lectures - Cell Image Classification: Methods and Applications
State-of-the-Art Lectures - High Parameter Flow Cytometry and a New Computational Platform Reveal Unique Cell Phenotypes that Predict Melanoma Outcomes
Plenary Session 1 - 3D Cytometry: Intelligent Image - Activated Cell Sorting
Plenary Session 1 - 3D Cytometry Quantitative Phase Imaging and Artificial Intelligence: Label-Free 3D Imaging and Analysis of Individual Cells
-
Contains 3 Component(s), Includes Credits Recorded On: 06/22/2019
A CYTO 2019 State-of-the-Art Lecture Presented by P. Chattopadhyay, D. Woods, A. Laino, S. Advani, V. Rao, A. Winters, J. Alexandre, & J. Weber
The Presenters
P. Chattopadhyay, D. Woods, A. Laino, S. Advani, V. Rao, A. Winters, J. Alexandre, and J. Weber
NYU Langone Health and RocketML, United StatesSession Summary
For patients with metastatic melanoma, immunotherapies have revolutionized treatment, but many patients fail to respond and mechanisms of resistance remain unclear. Moreover, many immunotherapy modalities are in clinical trials, but there are few biomarkers known to predict response. We believe a major reason is the lack of in-depth immune profiling, and a lack of tools that completely and comprehensively examine datasets. The work presented here represents a new approach to biomarker research, which combines 30-parameter flow cytometry (HPFlow) with CytoBrute, a rapid computation platform that performs high-parameter Boolean analysis that otherwise might be intractable. We tested PBMC from a clinical trial in which metastatic melanoma patients received different sequences of nivolumab (anti-PD1) and ipilumamab (anti-CTLA4). One sequence of drugs was more effective, but both cohorts had treatment responders and disease progressors. Using new HPFlow, we tested patients (n=35) at baseline, week 13 (between drugs), and week 25. We designed five 22+ color panels, which measured immune checkpoints, checkpoint ligands, T-cell maturity, transcription factors, and activation. In lieu of dimension-reduction (like t-sne) to analyze the data, we designed CytoBrute to compute the percent of cells expressing every possible combinations of markers, from single markers to combinations of two, three, and beyond. CytoBrute is fast; it reduces a process that takes four hours per file using R down to just 7 seconds. We found several phenotypes whose frequency at baseline was associated with response to each drug sequence. These included simple ones, like CD4+ CD95+ T-cells, which were higher at baseline in people destined to progress (p=0.0048). We also found various resting memory CD4+ T-cells (defined by simple sets of two markers) were elevated in individuals destined to progress, and this relationship was unique to one of the drug sequences. The fact that different markers for similar cells shared this relationship supported our interpretation. We also found a complex phenotype (defined by fourteen markers) which, when present at low frequencies, predicted a favorable survival profile (50 percent of patients were alive beyond 40 months after diagnosis, p=0.00095). In contrast, 50 percent of patients with high levels perished in ten months. We also found a new subset of regulatory T-cells that was important in responses, expressing suppressive markers like GARP and CD39 but not classical regulatory markers like CD25 and FoxP3. Finally, we integrated CytoBrute into the machine learning algorithm LASSO, identifying specific PD1+ subsets that were completely abrogated with one regimen, but not the other. This result provided the first insight into specific subsets that might be preferentially lost with that immunotherapy regimen. The machine learning algorithms generated models that could predict outcomes with area-under-the-curve values from 0.7 to 0.8, rivaling biomarkers currently used in immune-oncology. Finally, we discriminated patients and healthy controls with great specificity and sensitivity (AUC = 0.96), establishing very clear differences in immune composition even in the peripheral blood of melanoma patients. This supports the feasibility of blood-based immune monitoring (rather than invasive tumor-based analysis). Collectively, we have demonstrated a novel and powerful approach to interrogating complex immunophenotypes, which has great potential for biomarker research.
CMLE Credit: 0.5
-
Contains 3 Component(s), Includes Credits Recorded On: 06/22/2019
A CYTO 2019 State-of-the-Art Lecture presented by Gustavo Rohde, University of Virginia School of Medicine
The Presenter
Gustavo Rohde
University of Virginia School of MedicineSession Summary
Cell image classification methods are currently being used in numerous applications in cell biology and medicine. Applications include understanding the effects of genes and drugs in screening experiments, understanding the role and sub-cellular localization of different proteins, as well as diagnosis and prognosis of cancer from images acquired using cytological and histological techniques. We review three different approaches for cell image classification: numerical feature extraction, end-to-end classification with neural networks, and transport-based morphometry. In addition, we provide comparisons of four different cell imaging datasets to highlight the relative strength of each method.
CMLE Credit: 0.5
-
Contains 3 Component(s), Includes Credits Recorded On: 06/22/2019
A CYTO 2019 State-of-the-Art Lecture Presented by Atul Butte, University of California, San Francisco Keywords: Precision immunology, Color Wheel, Panel Design, CYTOBrute, Clustering, Immunotherapy, Nivolumab, Ipilimumab, Machine Learning, Elastic Net
The Presenters
A. Butte
University of California, San FranciscoSession Summary
There is an urgent need to take what we have learned in our new “genome era” and use it to create a new system of precision medicine, delivering the best preventative or therapeutic intervention at the right time, for the right patients. Dr. Atul Butte's lab at the University of California, San Francisco builds and applies tools that convert trillions of points of molecular, clinical, and epidemiological data—measured by researchers and clinicians over the past decade and now commonly termed “big data”—into diagnostics, therapeutics, and new insights into disease. Several of these methods or findings have been spun out into new biotechnology companies.
Dr. Butte, a computer scientist and pediatrician, will highlight his lab’s recent work, including the use of publicly-available molecular measurements to find new uses for drugs including new therapies for autoimmune diseases and cancer, discovering new druggable targets in disease, integrating, and reusing the clinical and genomic data that result from clinical trials, and how the next generation of biotech companies might even start in your garage. In particular, Dr. Butte will describe the public data resources in the NIAID ImmPort available to study immunology, infection, vaccination, and transplantation, and how these resources can be used to better target drugs and understand immunity across tens of thousands of individuals.
CMLE Credit: 0.5
-
Contains 3 Component(s), Includes Credits Recorded On: 06/22/2019
A CYTO 2019 Scientific Tutorial Presented by John Nolan, Josh Welsh, & Joanne Lannigan Keywords: extracellular vesicles, swarm, coincidence; MIFlowCyt; MIFlowCyt-EV; refractive index; controls
The Presenters
J. Nolan, J. Welsh, and J. Lannigan
Scintillon Institute, Center for Cancer Research, and University of Virginia, United StatesSession Summary
In this tutorial, we will review the objectives and challenges for measuring EVs by flow cytometry and present a framework and guidelines for reporting EV FC methods and results.
Tutorial Description
Extracellular vesicles (EVs) are of interest because of the wide range of potential roles they can play in normal physiology and disease. Their heterogeneity in biofluids motivate single particle analysis approaches such as flow cytometry, but their small size makes them difficult to measure, producing a literature that is rife with artifacts and irreproducible results. In order to promote improved rigor and reproducibility of EV FC measurements, a Working Group of researchers from ISAC, the International Society for Extracellular Vesicles (ISEV), and the International Society for Thrombosis and Hemostasis (ISTH) is developing a framework and guidelines for the reporting of EV FC methods and results. In this tutorial we will review this framework and the current state of reporting guidelines, with examples and suggestions for best practices that might improve the values of FC-based measurement of EVs.
CMLE Credit: 1.5
-
Contains 3 Component(s), Includes Credits Recorded On: 06/22/2019
A CYTO 2019 Scientific Tutorial Presented by Gustavo Rohde & M. Shifat-E-Rabbi Keywords: Microscopy, advance analysis, software analysis, image cytometry analysis, machine learning, visualization
The Presenters
G. Rohde and M. Shifat-E-Rabbi
University of Virginia, United StatesSession Summary
Cell phenotypes carry valuable information of biological processes. Cell image classification methods can help decode these complex processes and provide scientific insights. These methods can be used in numerous applications in cell biology and medicine, including understanding the impacts of genes or drugs, learning the roles subcellular proteins or staining patterns, as well as diagnosis and prognosis of certain diseases.
This tutorial will describe and compare three main approaches currently in use for classifying images of cells:
- Numerical feature extraction methods. We will describe how simple features (e.g., area, perimeter, and texture features) can be informative regarding cell state.
- End-to-end neural network models. We will describe how recent neural-network technologies (e.g., convolutional neural networks) can be used to classify images of cells.
- Transport-based morphometry. We will describe how image representation methods based on the mathematics of optimal transport can be used to classify images of cells while at the same time providing useful visual information regarding differences in classes.
The tutorial will also compare these different approaches on different publicly available datasets in order to highlight the relative strengths of each method. Finally, software implementing all of the strategies above will be described and distributed to participants. After participating in this tutorial, the students should have a comprehensive understanding of the importance of cell image classification, as well as the strengths/weaknesses of three main cell image classification approaches. They should also learn how to use the software to classify different cell images.
CMLE Credit: 0.5
-
Contains 3 Component(s), Includes Credits Recorded On: 06/22/2019
A CYTO 2019 Scientific Tutorial Presented by Slawomir Piatek, James Butler, & Earl Hergert Keywords: fluorescence, diffraction, refraction, voltage pulse, photosensitivity, dark current, noise, dynamic range
The Presenters
S. Piatek, J. Butler, and E. Hergert
New Jersey Institute of Technology and Hamamatsu Corporation, United StatesSession Summary
In flow cytometry, the light scattered off an interrogated cell contains information about the cell. The role of a photodetector is to transform this information from light signal to electrical signal. This task makes a photodetector an indispensable component of a functioning flow cytometer. Transformation of information from one domain to another is never lossless. A photodetector, together with the front-end electronics, will always introduce some degree of noise and signal distortion, which impacts ubiquitous-to-flow-cytometry scattered plots. Absent of a perfect photodetector, a practitioner can choose between devices such as a photodiode, avalanche photodiode, photomultiplier tube, or silicon photomultiplier. However, each of these has unique opto-electronic and performance characteristics; therefore, the optimal performance—the smallest impact on the scatter plot—should be the guiding principle in the selection process of the photodetector. The workshop discusses the operation and performance of each device, emphasizing topics such as intrinsic gain, sources of noise, detection bandwidth, and, most importantly, how these affect the scatter plots.
CMLE Credit: 1.5
-
Contains 3 Component(s), Includes Credits Recorded On: 06/22/2019
A CYTO 2019 Scientific Tutorial Presented by Geoffrey Lyon, Steve Perfetto, & Evan Jellison Keywords: aerosol testing, risk assessment, Glo-Germ testing, UV-aerodynamic particle sizer, YG beads, Cyclex-D test, Dragon Green Beads, aerosol evacuation system, SOPs
The Presenters
Geoffrey Lyon
Yale University School of MedicineSteve Perfetto
NIAID, National Institute of HealthEvan Jellison
UCONN HealthSession Summary
This tutorial will provide a summary of biosafety principles as they apply to flow cytometry and cell sorting, including a comprehensive review of an improved bead-based containment assay presented in paper “Novel Impactor and Microsphere-Based Assay Used to Measure Containment of Aerosols Generated in a Flow Cytometer Cell Sorter” (Perfetto. S., Cytometry A 2019; 95A: 173-182). This tutorial will also provide an expert forum highlighting specific scenarios that operators or core facility managers encounter. After this tutorial, the attendee should have a clearer understanding of the principles and practices of biosafety as they pertain to flow cytometry, in particular cell sorting. Additionally, the attendees will have an understanding of how to properly conduct this new bead-based assay to validate the containment of cell sorters.
CMLE Credit: 1.5
-
Contains 3 Component(s), Includes Credits Recorded On: 06/22/2019
A CYTO 2019 Scientific Tutorial Presented by Yvan Saeys, Ghent University—VIB Belgium
The Presenter
Yvan Saeys
Ghent University - VIB
BelgiumSession Summary
In this tutorial we will provide the students with some fundamentals about the different types of data mining techniques that can be applied to extract knowledge from single-cell data. In addition to the basic methodology, we will also give some examples of the different tools that implement these methodologies. Use cases of the different data mining methodologies will be illustrated on different types of single cell data: flow/mass cytometry, single-cell imaging, and single-cell transcriptomics data.
The following topics will be covered in the course:
- Data pre-processing and quality control.
- Overview of the different types of models (descriptive and predictive models).
- Techniques for automated gating (unsupervised and supervised techniques).
- Comparing samples Identifying biomarkers.
- Visualization of high-dimensional single-cell data.
- Modeling cell developmental trajectories.
At the end of the tutorial, the student should have a broad overview of the different types of data mining techniques that can be used to answer specific biological questions about his/her single cell data, as well as the tools that are around to perform these analyses.
CMLE Credit: 1.5
-
Contains 3 Component(s), Includes Credits Recorded On: 06/22/2019
A CYTO 2019 Scientific Tutorial Presented by Rob Salomon, University of Technology Sydney & Thomas Ashhurst, Centenary Institute Keywords: scRNA-seq, single cell technologies, data analysis, CITE-Seq, AbSeq
Presenters
Rob Salomon
University of Technology SydneyThomas Ashhurst
Centenary InstituteSession Summary
This tutorial will begin by explaining why the emerging field of genomic cytometry (the measurement of cells using genomic techniques), in conjunction with more traditional cytometry techniques such as fluorescence, mass, and imaging cytometry, is becoming a standard tool for biologists looking to unravel complex cellular processes and to develop a deeper understanding of heterogeneity. We will give a detailed overview of the various technologies that have allowed the emergence of genomic cytometry as well as those that continue to push the boundaries of cellular characterization. We will then provide a basic overview of the sequencing process such that both research cytometerists and the staff for the cytometry SRL are better equipped to understand the downstream genomic component of genomic cytometry. Finally, we will wrap up the session with case studies that illustrate the power of the genomic cytometry approach and will give a brief outline of where we feel the field needs to go as it matures. We expect attendees will gain a better understanding of 1) the rapidly maturing field of genomic cytometry and, 2) how genomic cytometry should be leveraged into more traditional cytometry workflows.
CMLE Credit: 1.5
-
Contains 3 Component(s), Includes Credits Recorded On: 06/22/2019
A CYTO 2019 Scientific Tutorial Presented by Bartek Rajwa, Purdue University & David Novo, De Novo Software Keywords: spectral cytometry, data analysis, unmixing, math, data processing
About the Presenters
David Novo
Dr. David Novo is the president and the founder of De Novo Software Inc. De Novo Software produces FCS Express, a comprehensive, robust, and easy-to-use flow and image cytometry data analysis platform. David is a member of the ISAC Data Standards Committee and frequently consults on data analysis issues related to flow cytometry. He collaborates on many projects involving novel data analysis requirements.
Bartek Rajwa
Dr. Bartek Rajwa is a research associate professor of computational life sciences in the Bindley Bioscience Center at Purdue University. He conducts studies on the technology of high-throughput cytometry, high-content imaging, biological image analysis, biological pattern recognition, and applications of statistical machine learning in cell biology. Bartek is an associate editor of Cytometry Part A and a member of the Board of Directors for the Society of Biomolecular Imaging and Informatics (SBI2).
Session Summary
The goal of this tutorial is to present and explain the mathematical underpinnings and the physical constraints of the spectral data analysis techniques commonly employed in the fields of multidimensional flow and image cytometry. The instructors will reintroduce and thoroughly review the concepts of photon-counting uncertainty, Poissonian, sub-Poissonian, super-Poissonian statistics, spectral overlap, linear mixing models, compensation, and spectral unmixing, as understood and applied in the context of polychromatic flow cytometry, multispectral flow cytometry, and modern spectral imaging microscopy.
The tutorial will also compare and contrast the conventional compensation paradigm with the more advanced unmixing approaches, focusing on how the implicit assumptions regarding noise may produce sub-optimal results. The material will cover the impact of noise and uncertainty on collected spectral data and, consequently, on subsequent modeling, analysis, visualization, and interpretation of unmixed results. The tutorial will describe and illustrate the most commonly utilized approaches to spectral data analysis, ranging from the basic unconstrained linear unmixing with least squares minimization to blind unmixing using a family of non-negative matrix factorization heuristics. The presentation will also briefly explore the applicability of principal component analysis and other dimensionality-reduction techniques for the exploratory preview of spectral data. The intended audience includes flow and image cytometry practitioners working with all types of optical single-cell analysis platforms such as traditional polychromatic cytometers, new multispectral machines, and various spectral imaging instruments. The tutorial assumes an intermediate level of understanding of modern cytometry techniques, as well as a basic knowledge of optics and photonics.
Tutorial Outline
- Basic principles of light.
- Brief introduction to fluorescence spectroscopy.
- Photon-counting statistics, photon interactions, photon collection, shot noise Poissonian, super-Poissonian, and sub-Poissonian light.
- Theory of photodetection PMTs, APDs, CCDs.
- Multispectral data-acquisition platforms in flow cytometry and imaging.
- Linear spectral mixture analysis (LSMA).
- Spectral overlap and linear mixing model.
- Use of compensation in polychromatic cytometry.
- Use of unconstrained LSMA in multispectral and hyperspectral systems.
- Abundance and non-negativity-constrained LSMA.
- Noise models in LSMA.
- Blind signal unmixing.
- Spectral data visualization and dimensionality reduction.
- Questions and open discussion.
CMLE Credit: 1.5